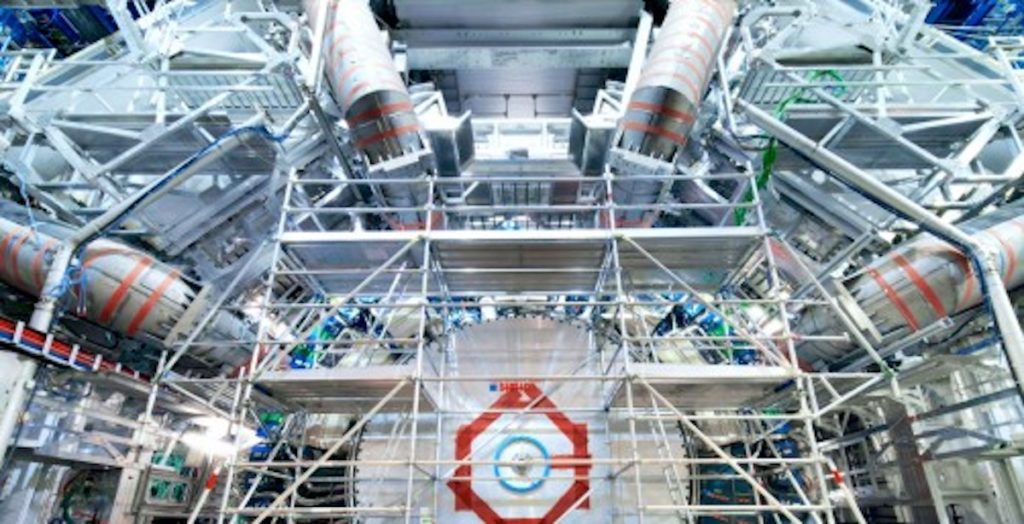
Mega Doctor News
In physicists’ ongoing search for new particles, it’s always easiest to find them when you have an idea where to look. Theories can serve to narrow and focus those searches to particles with a particular mass or a mass range, or to those with specific parent, child, or sibling particles as they burst forth from the firework-like cascades of particle collisions.
Far more challenging – like searching for a home on a map when you don’t know the address or the owner’s identity – is to search for a new particle without any clue of what the particle might look like or how it will behave.
But the effort is worthwhile to rule out particle hiding places, said Ben Nachman, a physicist at the U.S. Department of Energy’s Lawrence Berkeley National Laboratory (Berkeley Lab) who participated in a study that utilized artificial intelligence to scan for new particles in three years of particle-collision data from CERN’s ATLAS detector.
“What worries me is that maybe we’ve been looking in the wrong place, and that’s why we haven’t found any evidence of new physics yet,” said Nachman, who participates in CERN experiments as a member of the ATLAS group at Berkeley Lab. “Our main motivation is that we do a lot of searches focused on a particular model. This new approach is in a class that’s model-agnostic.”
While the entire ATLAS collaboration contributed to the latest result, Nachman conceived of the analysis and led the effort, along with Berkeley Lab affiliate and SLAC National Accelerator Laboratory-affiliated postdoctoral researcher Aviv Cukierman.
The new exploration, detailed in the journal Physical Review Letters, relied on machine-learning algorithms to search for anomalies in ATLAS detector data collected from 2015-18, without relying on any particle simulations. Machine learning is a form of artificial intelligence that can improve its searches over time as it is fed more data.
In the study, the researchers trained machine-learning algorithms on actual data for particle-collision events involving two “jets,” which are narrow cones of particles produced in collision events that tend to travel in the same direction through the detector. To manage the massive amount of data, researchers also set a minimum energy threshold for their search.
The algorithms were set loose on a “bump hunt,” which is a search for any unexpected, statistically significant signals at any specific energy. It was designed to be sensitive to particle events including those in which an unknown particle decayed into two other unknown particles – such a search had never before been conducted using ATLAS data.
“This first test didn’t find any evidence for new particles,” Nachman noted, “but for a given set of models we show that we have broader coverage than any previous searches – we cast a big net. And we have a lot of sensitivity.” That big net included the use of about 20,000 neural networks, which are sets of algorithms that are used in the machine-learning training process.
Part of the challenge of the study was in identifying which neural networks were the most likely to succeed in detecting anomalies. “We can’t investigate all 20,000,” he said.
Researchers noted in the study that the neural networks were trained on simplified 2D space, represented by images, “so there is great potential to extend this method to include additional features and more final states in order to ensure broad coverage of unanticipated scenarios.”
Nachman said he’s looking forward to casting an even wider net using Perlmutter, a supercomputer being installed at Berkeley Lab’s National Energy Research Scientific Computing Center (NERSC). Perlmutter is equipped with graphics processing units (GPUs) and can implement more sophisticated machine-learning algorithms on the ATLAS data.
“We will have tons of GPU nodes to take this analysis to the next level,” he said.
NERSC is a DOE Office of Science user facility. The ATLAS Collaboration is supported in the U.S. by the U.S. Department of Energy’s Office of Science and the National Science Foundation.