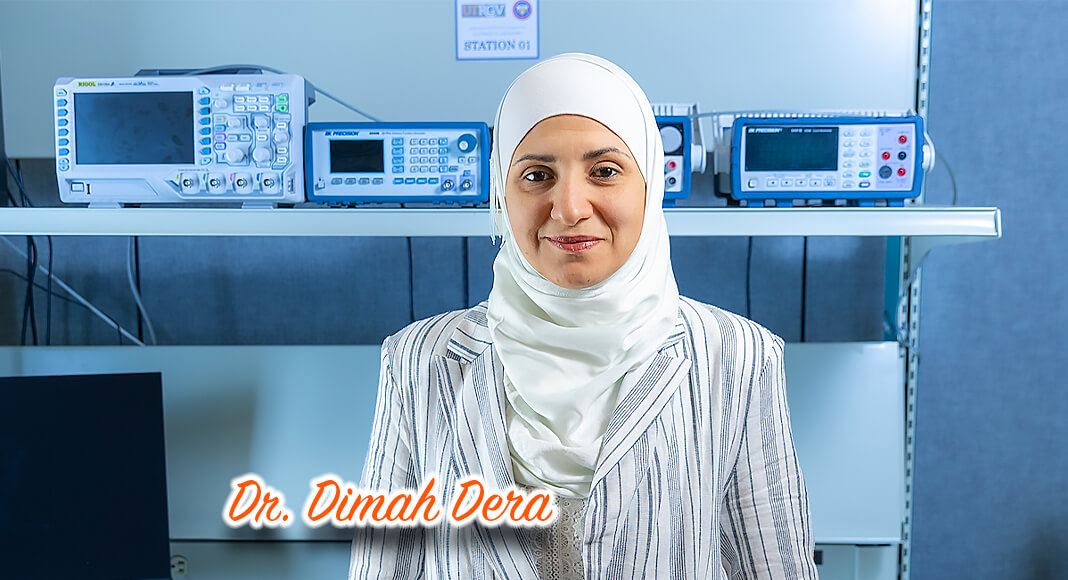
Mega Doctor News
By Karen Villarreal
RIO GRANDE VALLEY, TEXAS – The likelihood of developing a cancerous brain tumor in your lifetime is less than one percent, but if you did, you would rely heavily on your doctor’s ability to identify the mass from MRI scans.
Currently, physicians spend considerable time manually detecting the size and shape of tumor structures, which can delay detection of tumor growth and result in an increase of tumor volume.
To facilitate that identification process, Dr. Dimah Dera, PhD, assistant professor in the UTRGV Department of Electrical and Computer Engineering, is part of a team developing an artificial intelligence-based system that automatically will identify tumor boundaries and volume.
“Brain tumor patients have to take regular scans to see how the tumor changes over time, and each MRI appointment results in over 400 images that have to be manually reviewed,” Dera said. “It can be very time consuming for their doctors, and time can be critical.”
To address the problem, Dera and two students, along with her collaborator, Dr. Nidhal C. Bouaynaya from Rowan University in New Jersey, have established a machine-learning AI algorithm that detects and delineates tumors based on a catalogue of real-world MRI scans.
A student from each university is working on the project through its completion in Spring 2023. Giuseppina Carannante, a PhD candidate at Rowan University, and Fazlur Rahman Bin Karim, a UTRGV master’s student in the mechanical engineering graduate program, are on schedule to graduate that semester, as well.
The software in development – designed to automatically delineate brain tumors – can be integrated into hospitals to detect tumors earlier and monitor progression of cancer or the success of the treatment success. And that gives doctors more time to spend with their patients.
Though the algorithm is developed to identify brain tumors, Dera said, the work could be extended in the future to detect the size of tumors anywhere in the body.
“It will save doctors time, and save their patients lots of suffering,” Dera said.
THE BIG PICTURE
According to the American Society of Clinical Oncology (ASCO) website,Cancer.net:
- Brain cancer is the 10th leading cause of death for men and women. The five-year survival rate in the United States for people with a cancerous brain tumor is almost 36 percent, and the 10-year survival rate is almost 31 percent.
- Around the world in 2020, an estimated 251,329 people died from primary cancerous brain tumors.
- It is estimated that 25,050 adults (14,170 men and 10,880 women) in the United States will be diagnosed with brain cancer in 2022, and that 18,280 adults will die from it.
- 1,957 new cases of cancers that start in the brain are expected to be diagnosed in Texas in 2022, with 1,342 Texan deaths expected from the disease.
IDENTIFYING A PROBLEM
Dera was motivated to develop the software during her doctoral program after choosing to dedicate her research to biomedicine. Working on a project with Bouaynaya at Rowan University and Dr. Hassan M. Fathallah-Shaykh, PhD, MD, a neurologist at the University of Alabama School of Medicine, brought the need for an AI system to her attention.
“Part of Fathallah-Shaykh’s work was to review MRI images and administer radiation therapy based on the observed growth,” she said. “He explained that there is no automatic computer-assisted software or system in use at hospitals.”
With Artificial Intelligence (AI) systems – which are based on data-driven algorithms – becoming increasingly popular during her post-doctoral studies, Dera spied a potential solution to the problem.
“These algorithms are being advanced for deployment in the medical field because of their high performance and accuracy,” she said. “I started looking at how we can integrate that to analyze radiological images and develop a new, robust tumor detection software.”
With her background in mathematics and computer engineering, she started building a mathematical model, but noticed the model’s limitation in handling a variety of tumor shapes and structures.
“Brain tumor segmentation is a challenging problem due to the complex topology of anatomical structures,” Dera said. “Tumors vary from one patient to another.”
Furthermore, doctors and the AI alike face “noise” during image acquisition – that is, unclear pictures due to the limitations of the MRI.
In February 2021, Dera received a $35,000 research grant from NJ Health Foundation, with $9,000 shared with Rowan University. Now, she and her collaborators are making progress on the project, entitled “Towards Robust Brain Tumor Detection and Surveillance.”
INCORPORATED SAFETY DETECTION
Dera said that, prior to writing the code, theyinterviewed neurologists through NSF’s Innovation Corps (I-Corps™) program – which is designed to help move research from the academic to industrial integration. They further identified neurologists’ needs and goals in reviewing the MRIs.
Dera said one problem in the field of medical technology is that human lives are in the hands of software – which can make mistakes and can be susceptible to attacks from computer viruses.
“We’re talking about human health, so we need to know how much we can trust the algorithm,” she said. “Mistakes could cause death and other consequences for the patient, and there’s always a margin of error.”
To address those weaknesses, the research team is developing a way for the algorithm to report on its own confidence with each image it analyses – which ensures that it is detecting real tumors, and not “noise” or artifacts from the imaging process.
The team also is incorporating “failure mode” detection systems, so that if the AI is attacked by a virus, it will determine if the virus has damaged its code and caused it to make incorrect decisions about the tumor’s boundaries or volume.
“Trustworthiness is the critical point in medical imaging,” Dera said.
REAL-WORLD APPLICATION
Dera’s grant funding was for one year, which began in Spring 2021, and she has secured an extension until Spring 2023.
After developing the mathematical foundations of the algorithm and validating it on synthetic and real, publicly available data sets from MRI images, Dera predicts they will complete their part of the project – developing a working algorithm– within the time allotted.
Then, they will hand it over to an independent startup company calledMRI Math, LLC., to integrate the code’s final user interface into a hospital’s private software system.
“We will meet with neurologists, hospital technologists and radiologists before that point to ensure that our code meets their needs,” Dera said.
Hospital systems are complicated, she said, so the team is not handling any aspect of the integration.
“The hospital-specific code is private,” she said. “That includes the user-interface design and other functionality specific to their software.”
OPEN-SOURCE AI
Although the hospital will be the sole owner of their integrated software, the foundational source code will be publicly available upon completion of the project, Dera said.
They’ve submitted some results to an engineering and medical imaging journal, IEEE Transactions on Medical Imaging, to get feedback from the scientific community and share what they’ve discovered. She hopes to hear back on that review by the end of summer 2022.
“Sharing our efficient implementations of the proposed algorithms through journals not only gives us feedback, but also benefits other researchers and allows them to build on our work,” she said.
And, by making the algorithm open-source, anyone interested can apply it to other projects, or experiment by running the code on publicly available images.
“This is how we advance in research. It’s good for the research community to make this work available,” Dera said. “That’s what we believe in.”